|
 |
 |
 |
 |
 |
 |
 |
 |
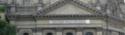 |
 |
 |
 |
 |
 |
 |
 |
 |
 |
 |
|
 |
 |
|
Modeling of Supercritical Fluid Extraction by Artificial Neural Networks
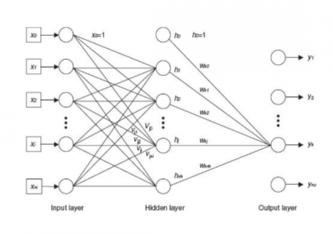
|
|
Separation processes play a crucial role in biomaterial processing. A gas when compressed
isothermally to pressures more than its critical pressure, exhibits enhanced solvent power
in the vicinity of its critical temperature. Such fluids are called supercritical fluids (SCF),
which possess specific characteristics that make them attractive as solvents. In particular,
solvent density, and hence solvent effectiveness, can be controlled by pressure and temperature.
Liquid-like densities and gas like viscosity, coupled with diffusion coefficients that are at
least an order of magnitude higher than those of liquids, contribute to enhancement of mass
transfer. The experiments to obtain high-pressure phase-equilibrium data are very difficult and
costly to conduct. Hence, it is extremely useful to develop a proper model or correlation
scheme to extend and complement the experimentally obtained data for SCF systems at high pressures.
Artificial neural networks are well known for their capacity to learn from real patterns and
to generalize the input-output relationship, especially for those high non-linear processes.
They have been widely used in task domains ranging from concept learning to function approximation.
In this project, an artificial neural network that considers the system as a black box is designed
for the mass transfer modeling of supercritical fluid extraction. In addition, a hybrid model
using both neural networks and the Peng-Robinson state equation is developed for supercritical
fluid extraction, where the neural network is used to generate the non-linear binary interaction
parameter of the Peng-Robinson state equation. The effectiveness of the proposed neural network
approaches are demonstrated by comparison studies. The results using the proposed models are better
than those using the conventional model.
|
|
 |
 | |
|